Why your world in business analytics is not flat
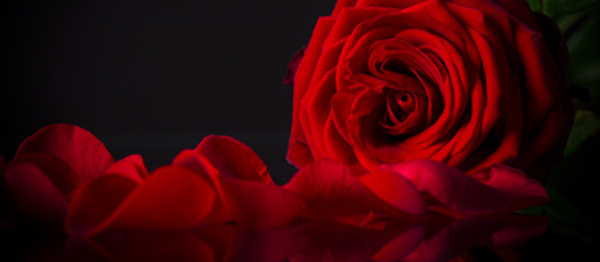
If you spot a red rose, smell on it and walk with your eyes over the details of its blossom, you will discover amazingly complex forms, colors, smells and surfaces. Although a red rose is highly complex, it is just beautiful. When we look at the picture of how things relate to each other, we rather prefer lines and squares. After 20 years data mining experience I can assure you: business reality does not consist of lines and squares at all. Instead it has great complexity and with it comes beauty.
Why spreading a few product samples may work and a lot don’t
Daniel was leading the commercial effectiveness department of a pharmaceutical corporation. His educational background as a molecular biology expert has made him skeptical about conventional modeling techniques that were promising to measure the ROI of his marketing and sales activities. “A houseplant has two success factors, water and sun” he said to me “if you put the plant in the dark basement, you can pour as much water as you like, without any positive impact”.
This is what conventional techniques neglect, they assume factors to be independent. If they don’t they assume something else. They assume lines or squares where reality holds beautiful curves. And this was why Daniel was calling me.
We collected the best sales and marketing data available. Beside sponsorships, local workshops, journal advertisement, brand reminder, direct marketing material, there was also the instrument of providing product samples. Conventional models would have indicated “yes, product samples works, the more the better”.
What we found looked beautifully complex and interesting, but odd at first sight. The relation had an inverted-u shape. At some point, providing sample did more harm than good. How can this be? Surely, every peace you provide to physicians will be handed over to patients. But physicians typically have limited patients. Too many samples do not stimulate but substitute prescriptions. That is how simple, plausible and beautiful real word complexity can be.
Why rebates sometimes work and sometimes don‘t
Recently, we dove into modeling success factors of sales in apparel stores. Two factors, “tangible rewards” and “good interpersonal communication”, seemed to be of major importance as standard multivariate models indicated. Conventional methods suggested “do both, the more the better”. What we found using advanced methods was much more beautiful, complex and meaningful. It turned out that tangible rewards only show impact if interpersonal communication is weak.
Reversely, improving interpersonal communication would already bring the highest return even when no tangible rewards were given to customers. Research in motivational psychology knows this phenomenon: extrinsic motivators like tangible rewards and intrinsic motivators does not add up, it can just substitute each other.
The recommendation to business is highly profitable: We found that training and choosing right staff is the lower investment compared to giving tangible rewards to customers at the long run. Indirect price discounts were prevented, sales margin grew by 2 percent bottom line.
When I present such examples to statisticians and other experts in quantitative reasoning they tend to say: “I can do the same with standard procedures”. What they usually do not consider is the fact that standard techniques can only model complexity (i.e. interactions and non-linearity), if the type and form of complexity is known upfront. But this is typically not the case in practice.
For instance, you need to know upfront that intrinsic and extrinsic motivators does not behave like water and sun for a houseplant (“AND” interaction) but is characterized by an “OR” interaction. In cases with 50 factors you then need to specify more than 2500 combinations and its types. What practitioner need in order to learn from data are methods that help to explore something new, something unexpected.
Fred Bookstein ones said at a scientific conference “A research method is valuable when it has the capacity to surprise with findings that hits you like a rake between your eyes”. This is exactly what I experience week by week.
Recently we were researching the top buying factor for a B2B production company. We asked the industry experts about the buying factors. However, as a kind of non-expert in this area, we felt uncomfortable with the list and added a few more items that are known from the literature. Then we studied which hidden factors truly drive decisions. Amazingly, two of the resulting top three drivers were not on the expert list. The business world is too complex, too beautiful, to purely rely on simple judgments.
We need to acknowledge that the world is complex like a beautiful blossom. Conventional statistics take a reductionist approach. They force data to be linear and simple and usually do not account for the data’s inherent complexity. Explore non-linearity’s and complex interactions without assuming things you don’t know. The insights will hit you like a rake between your eyes.
If you find this post useful, we appreciate if you “like” it and share it.